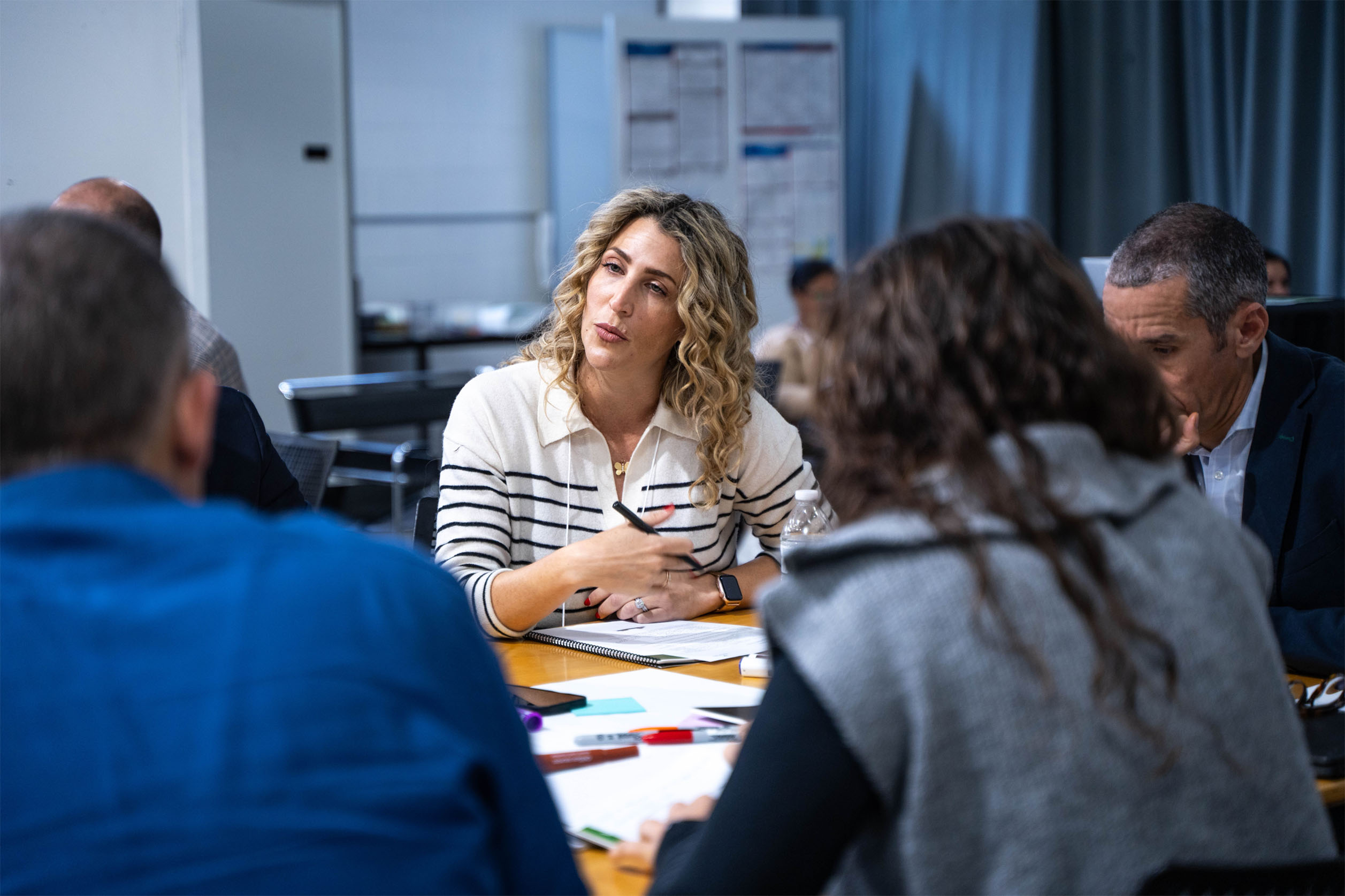
Although regulatory agencies recommend mitigating risk as much as possible through early revisions to the protocol and investigational plans, some safety and quality risks might still exist during study conduct. Therefore, risk-based monitoring (RBM) or study oversight plans are important to identify and address any unexpected issues that occur during the study. RBM typically uses a targeted, strategic approach to risk mitigation that relies on data review, analytics, and visualizations. Selecting the right statistical approach to effectively and quickly identify study-related issues helps minimize their effect on trial quality and has the potential to offer a compelling approach to drive efficiency and speed by prioritizing the clinical trial risks most associated with essential safety and efficacy data.
The following sections on statistical approaches summarize the information presented at the RBQM India Summit in June 2024 by Biswadhip Pai, Senior Manager of Risk-Based/Central Monitoring in Veranex’s Clinical Data Services.
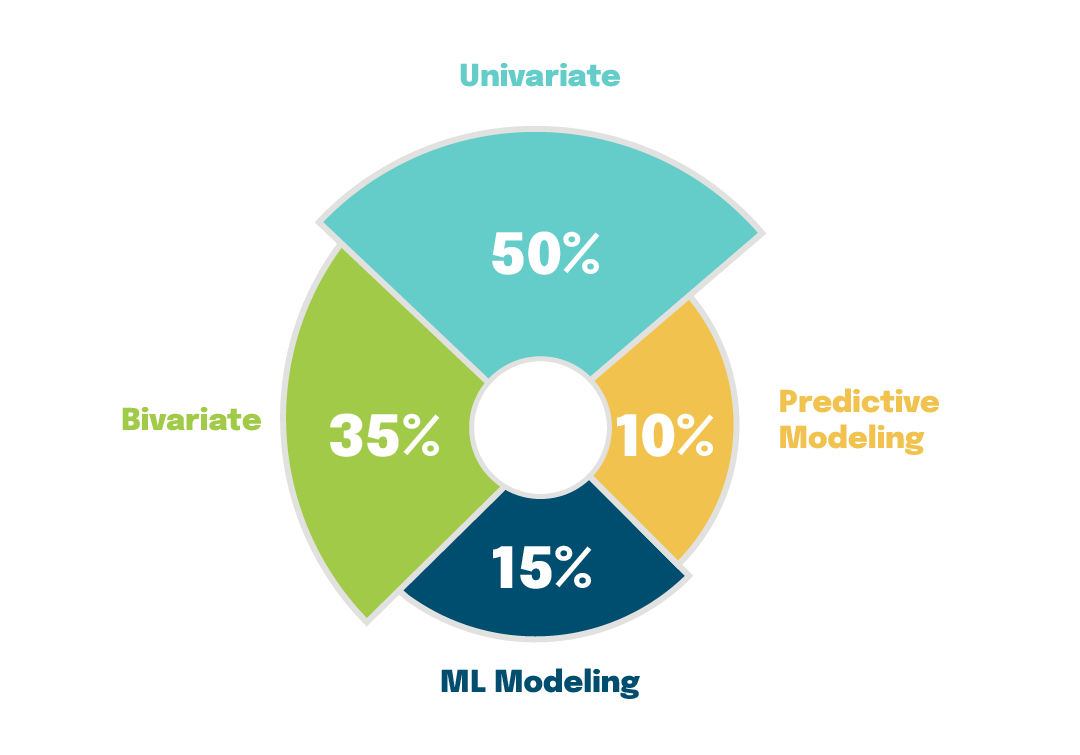
Figure 1 Use of different statistical approaches based on the trial data distribution
Statistical approaches
Statistical data monitoring involves the use of statistical tests on some or all of the study data to identify atypical data patterns at sites that may be the result of inaccurate recording, training issues, or study equipment malfunction or miscalibration. Although there has been a move in the industry toward data-driven technologies for RBQM, such as machine learning (ML) and predictive modeling approaches, their use is often not warranted for many trials. Instead, there remains a pressing need for efficient, simple, and easily interpreted RBM solutions that also have a high accuracy rate and reliably detect anomalies for use across most trials, such as univariate (e.g., visualizations via box-whisker plots, histograms, violin plots, control charts) or bivariate (e.g., determining relationships or correlations between variables) analyses. For example, when we look at the statistical approaches used in the 30+ studies using RBQM that Veranex has been involved with over the past six years, we see that, for approximately 50% of clinical trial data, a univariate approach was more informative for detecting outliers (Figure 1). For 35% of the data, a bivariate approach helped provide statistical insights. For only 15% of the data, an ML modeling approach (multicollinearity, heteroscedasticity, model parsimonious, model diagnostics such as the AIC and BIC) was effective, while predictive modeling approaches (accuracy, precision, recall, F1 score, confusion matrix, AUC) were only effective for presenting 10% of data.
Diving into univariate approaches
As the approach that applies to the majority of clinical trial data, univariate analysis deserves a deeper dive. First, there are a couple of key terms that should be defined: quality tolerance limits (QTLs), or “predetermined limits for specific trial parameters that, when reached, signal that further evaluation is needed to determine if action is warranted,” and key risk indicators (KRIs), or “metrics used to assess site performance, either compared with other sites or to established values.”
Univariate analyses help identify data anomalies, playing a key role in almost all study KRIs. Commonly used KRIs include the screen failure rate, serious adverse event (AE) rate, non-serious AE rate, early termination rate, missed assessment rate, eCRF visit-to-entry cycle time, protocol deviation rate, and off-schedule visit rate.
Viewing and understanding the data distribution via a simple control chart or box plot can aid in detecting outlying data that could signify an issue (Figure 2). Conducting data transformation (e.g., logarithmic or reciprocal) could increase the accuracy and effectiveness of outlier detection, prior to selecting the specific methodology. Finally, a consistent strategy using a site- or participant-level color-coded status of Red, Amber, or Green (RAG) facilitates easy visualization of values that might require additional attention.
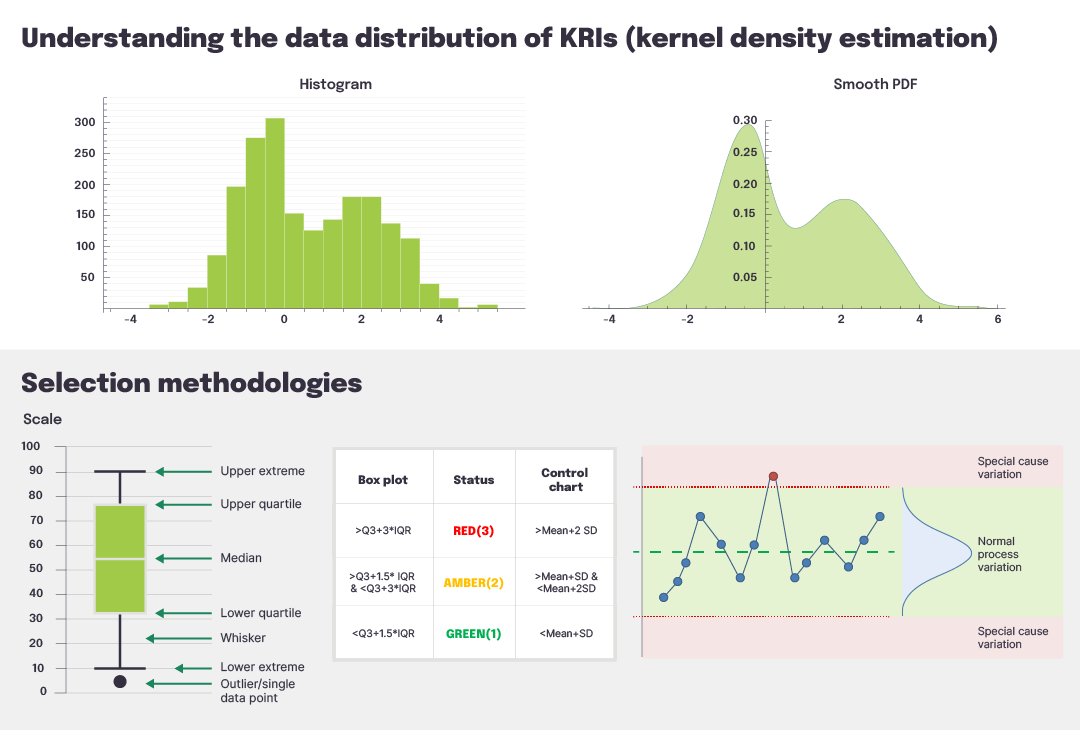
Figure 2 Understanding and classifying KRI distributions
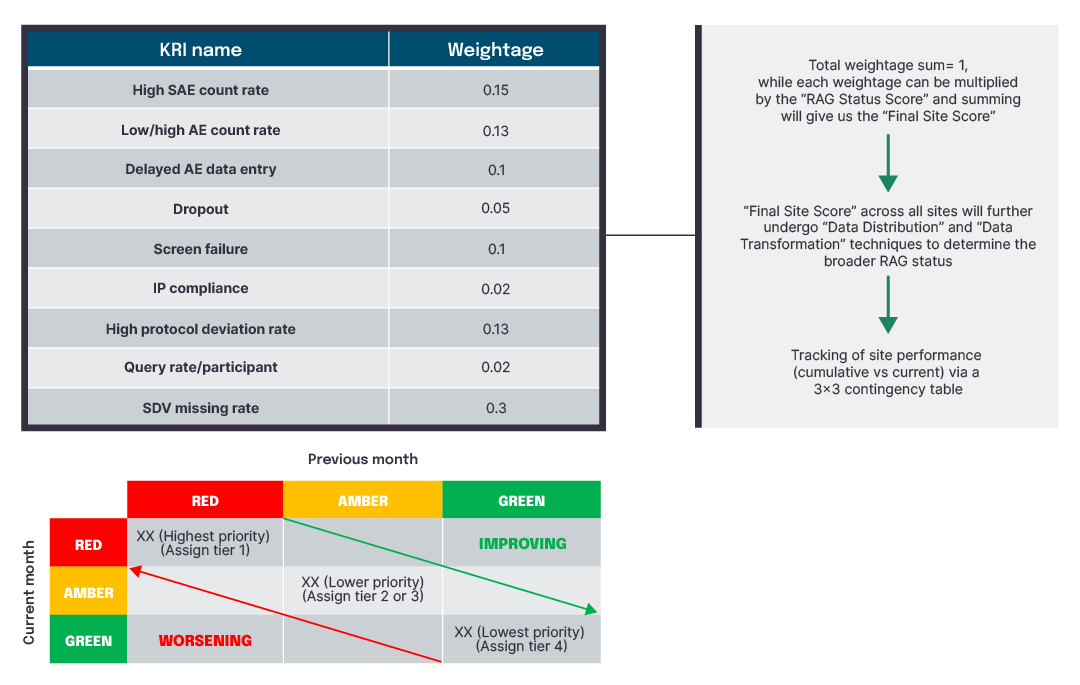
Figure 3 Site-level scoring using univariate analyses
Looking at trends across sites and countries via visualization dashboards facilitates easy recognition of areas for improvement that could impact trial quality (Figure 4). Further comparison of individual sites against study-level metrics is valuable for targeted resolution strategies such as additional site training or oversight (Figure 5).
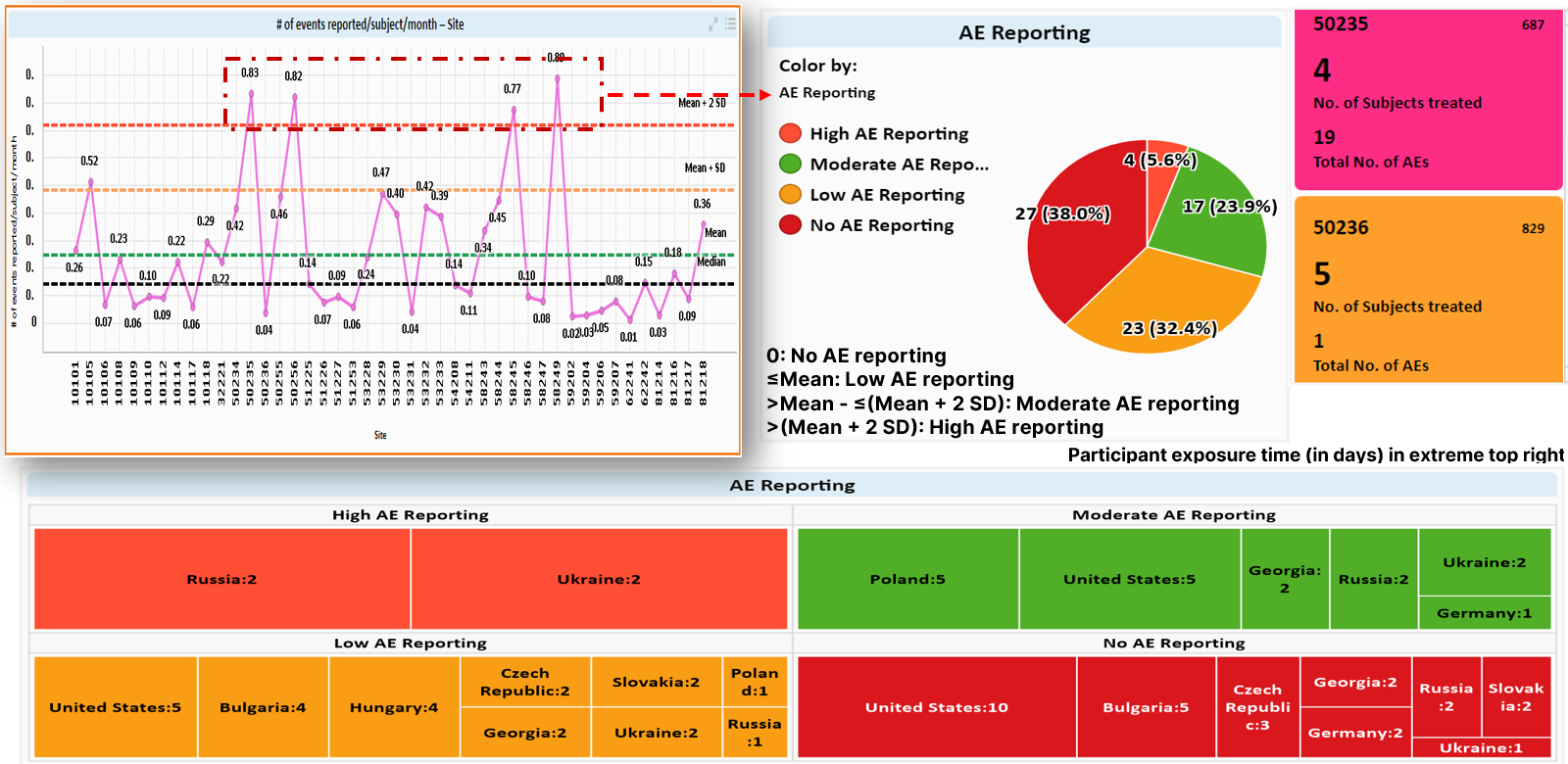
Figure 4 Example of univariate analyses of AEs by site and country
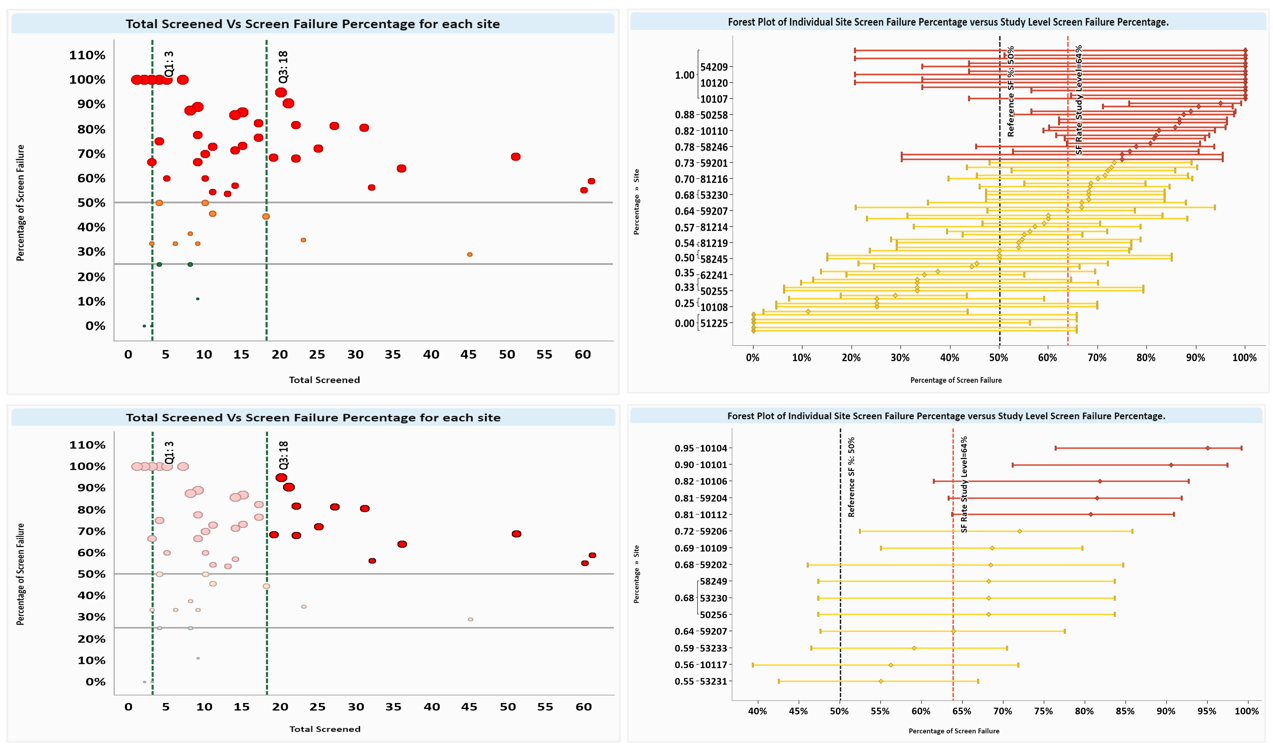
Figure 5 Example of screen failure analysis using a quadrant approach
RBQM: a dynamic process
For the best outcomes, a dynamic approach to RBQM is recommended. Periodic discussions between stakeholders complemented by a series of statistically driven steps can help assess the risk for any study protocol. These steps follow those recommended by regulatory agencies: risk identification, risk evaluation, risk control, risk review, and risk reporting. Collectively, they help determine how to minimize risks to participant safety, data quality, and CRA workload. Our Clinical Data Services teams are experienced at designing and implementing RBQM across a broad range of therapeutic areas in trials both small and large. Contact us to discuss how these strategies can be applied to your next study.