As artificial intelligence transforms healthcare innovation, the FDA continues to evolve its regulatory framework for AI-enabled device software functions (AI-DSF). In 2024-2025, the agency released two crucial guidance documents that reshape the submission process for medical device manufacturers.
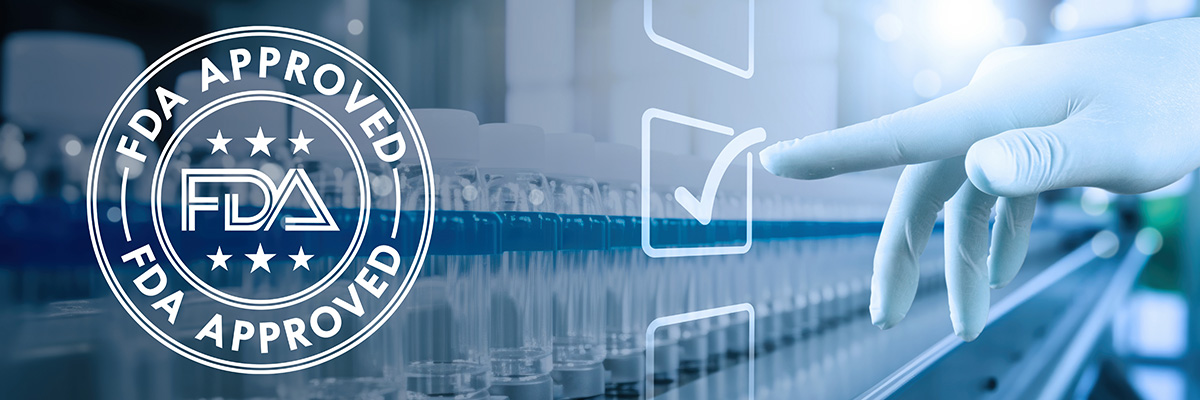
Understanding the New FDA AI Guidance Framework
The FDA has introduced two significant documents that provide comprehensive direction for AI/ML-enabled medical devices:
- FDA Artificial Intelligence-Enabled Device Software Functions: A draft guidance focusing on lifecycle management and marketing submission recommendations (January 2025)
- Marketing Submission Recommendations for AI/ML-Enabled Device Software: Specific guidelines for predetermined change control plans (December 2024)
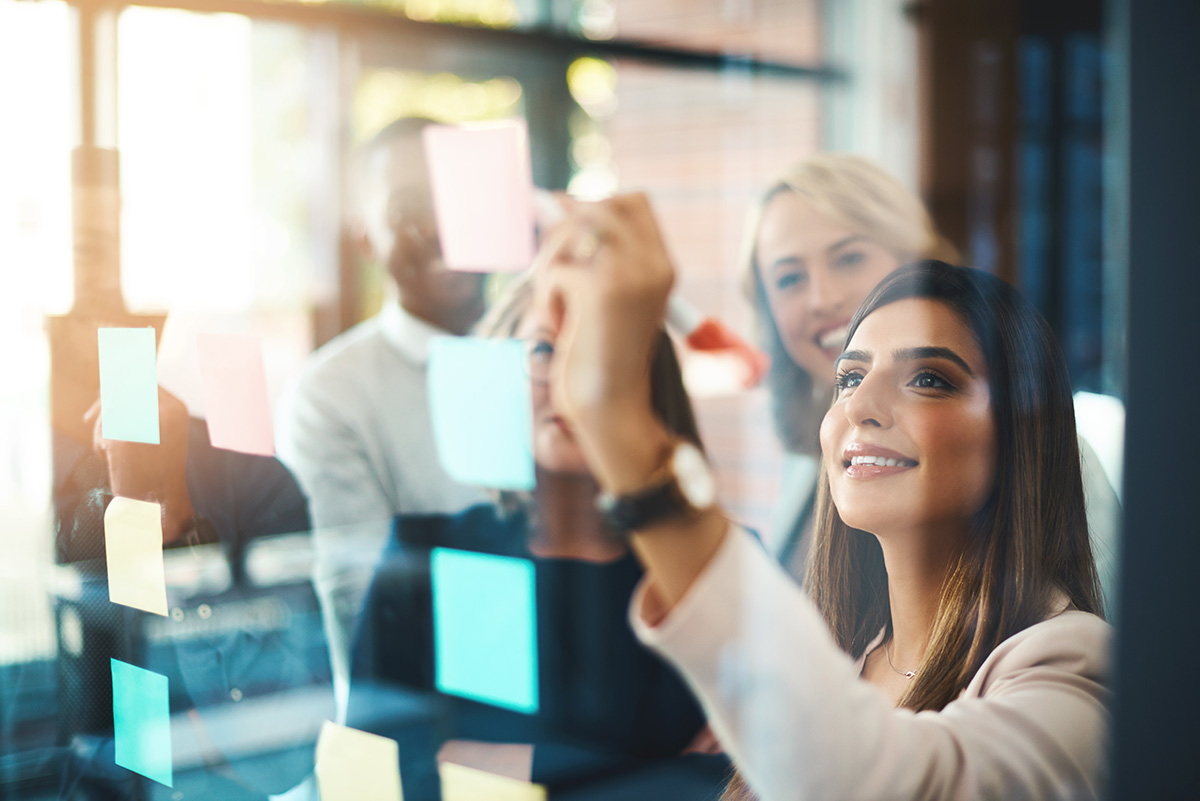
Our regulatory team has extensive expertise in navigating the submission process. If you have questions about your AI-enabled device, get in touch here.
Key Components for Marketing Submission Success
Early FDA Engagement Strategy
Why It Matters:
- Ensures alignment between development and FDA expectations
- Streamlines the review process
- Ensures the continued safety and effectiveness of a device without necessitating additional marketing submissions
- Critical for novel technologies and validation methodologies
Best Practices for Early Engagement:
- Schedule pre-submission meetings early in development
- Prepare comprehensive development and testing plans
- Document validation strategies thoroughly
Activities to Satisfy the Marketing Submission
This section outlines the steps and documentation recommended to prepare and submit your device for FDA marketing authorization.
A. Early Engagement with the FDA:
Purpose:
To ensure your development and testing plans align with the FDA’s expectations and to facilitate a smoother review process. This is particularly important for new and emerging technologies or novel validation methods.
Details:
- Identify the appropriate FDA review division: Determine which division within the FDA’s Center for Devices and Radiological Health (CDRH), Center for Biologics Evaluation and Research (CBER), or Center for Drug Evaluation and Research (CDER) is responsible for devices similar to yours. For combination products, engage with the lead review division or consult the Office of Combination Products (OCP) for lead review division assignment.
- Prepare a Pre-Submission (Pre-Sub) package: This package should include a detailed description of your device, its intended use, the AI/ML methodologies employed, proposed testing plans, and any specific questions you have for the FDA.
- Request a meeting with the FDA: Utilize the Q-Submission program to formally request feedback on your development plans and marketing submission strategy. This allows for valuable early interaction and clarification. The guidance emphasizes the importance of engaging early to ensure testing supports the total product lifecycle and a risk-based approach.
B. Comprehensive Documentation for Marketing Submission:
Purpose:
To provide the FDA with a thorough understanding of your device’s design, development, validation, and performance.
Details:
Organize your submission with clear and detailed documentation in the following areas:
Quality System Documentation:
Consider if the information required in the marketing submission can be sourced from your Quality System documentation. This can include documentation related to design validation, design changes, and handling of nonconforming products.
Device Description:
- State that AI is used in the device.
- Clearly define the intended use of the AI-enabled device including AI model features, user qualification, and compatible input devices and acquisition protocols.
- Describe how the AI component contributes to achieving the intended use.
- Detail the device architecture, including hardware and software components.
- Describe device inputs (manual or automatic) and outputs, including compatible input devices and acquisition protocols.
- Include a use environment and clinical workflow description.
User Interface and Medical Device Labeling:
- Describe the user interface and how users will interact with the AI-enabled features.
- Provide clear and concise labeling that includes information on how the AI helps achieve the device’s intended use, model architecture, model inputs and outputs, performance metrics and their monitoring, known limitations, and the potential for the model to change over time.
- Include information on installation and maintenance instructions, especially regarding integration with data systems and ensuring input data compatibility. Define terms explicitly (e.g., “sex”).
- Describe any customizable features, how to configure them, when it’s appropriate, and how users can discern the current configuration.
- Explain any additional metrics or visualizations used to add context to the model output.
- For devices intended for patients or caregivers, provide labeling material at an appropriate reading level, describing instructions for use, indications, intended use, risks, and limitations. Explain how patients/caregivers will understand device use and output if specific materials aren’t provided.
Risk Assessment:
- Provide a “Risk Management File” including a risk management plan, a risk assessment and a risk management report.
- Consider risks across the Total Product Life Cycle (TPLC) in both normal and fault conditions, including those resulting from user error.
- Specifically consider risks related to understanding information necessary to use or interpret the device, including risks related to lack of information or unclear information.
- Explain risk controls, including user interface elements (e.g., labeling).
Data Management:
- Provide a clear explanation of data management practices (i.e., collection, processing, annotation, storage, control, and use).
- Characterize the data used in development and validation, which is critical for understanding how the device was developed and validated.
- Describe data sources, characteristics, and preprocessing steps.
- Explain how data quality and representativeness are ensured, including addressing potential biases.
- Document data annotation methodologies and expertise involved.
- Describe data governance, security, and privacy measures.
- Explain data provenance and audit trails.
Model Description and Development:
- Provide a comprehensive explanation of the AI model(s) used, including the algorithms, architecture, and training methodology.
- Document the rationale for model selection and any design choices made.
- Describe the model inputs and outputs, including a description of any pre- or post- processing, and/or data augmentation or synthesis, as applicable.
- Explain the model architecture, including layers and connectivity.
- Detail the training process, including data splitting, augmentation, and optimization.
- Describe the performance metrics used during development and their target values.
- Explain the handling of uncertainty in model outputs.
- Describe the use of ensemble methods, if applicable.
- Explain how thresholds (operating points) were determined.
- Explain any calibration of the model output.
Validation:
- Provide comprehensive validation data demonstrating the safety and effectiveness of the AI-enabled device for its intended use.
- Include details on the validation datasets, methodologies, and acceptance criteria.
- Address the performance of the device across relevant demographic groups to mitigate bias.
- Include a software version history, detailing model versions and differences between tested and released versions.
Performance Validation:
Provide objective evidence that the device performs predictably and reliably in the target population.
- Describe the validation study design, including the rationale for the chosen approach.
- Characterize the validation dataset, ensuring it is appropriate for the intended use population and accounts for potential data drift.
- Define performance metrics and acceptance criteria, justifying their relevance.
- Present results for the overall population and relevant subgroups (stratification).
- Include uncertainty and variability analyses.
- Discuss clinical significance of the findings.
Human Factors Validation (or Usability Evaluation):
Demonstrate users’ ability to interact with and understand the device safely and effectively.
- Describe the methodology used for human factors validation or usability testing.
- Characterize the user groups involved in the testing.
- Present the results of the testing, focusing on the effectiveness of risk controls related to information understanding.
Medical Device Monitoring:
- Describe data collection and analysis methods for identifying and assessing post-market changes in model performance and their impact on safety and effectiveness.
- If employing proactive performance monitoring as a risk control, include information regarding your performance monitoring plans.
- Explain how you will monitor potential causes of undesirable performance changes (e.g., changes in patient demographics, data drift, user behavior).
- Describe robust software lifecycle processes for monitoring in the deployment environment.
- Outline your plan for deploying updates, mitigations, and corrective actions.
- Describe procedures for communicating performance monitoring results and mitigations to users.
Cybersecurity:
- Include elements in the cybersecurity risk management report, threat modeling, cybersecurity risk assessment, labeling, and other deliverables specified in the “Cybersecurity in Medical Devices: Quality System Considerations and Content of Premarket Submissions” guidance.
- Explain how cybersecurity testing addresses risks associated with the model, including malformed input (fuzz) testing and penetration testing.
- Provide a Security Use Case View covering AI-enabled considerations.
- Describe controls for data vulnerability and preventing data leakage (access controls, encryption, anonymization or de-identification of sensitive data).
- Consider controls for data poisoning, overfitting, model bias, and performance drift attacks (data validation, anomaly detection, adversarial training, differential privacy, secure multi-party computation, watermarking, continuous monitoring).
Public Submission Summary:
Prepare a summary document outlining the device’s intended use, key technological aspects, and performance data.
C. Addressing Transparency and Bias:
Purpose:
To ensure the FDA and users understand the AI’s functionality and to mitigate potential biases that could impact safety and effectiveness.
Details:
Transparency in Labeling:
Provide clear information in the labeling about the AI aspects of the device, including how AI is used, model inputs/outputs, performance metrics, and limitations.
Bias Mitigation:
- Ensure representativeness in data collection during development, testing, and post-market monitoring across all relevant demographic groups (e.g., race, ethnicity, sex, age).
- Collect evidence to evaluate whether the device benefits all relevant demographic groups similarly.
- Document the steps taken to identify and mitigate potential biases in the AI model and its data.
- Stratify performance validation results by relevant subgroups.
D. Predetermined Change Control Plan (PCCP) Submission (If Applicable):
Purpose:
To obtain premarket authorization for anticipated modifications to the AI-enabled device software function without requiring a new submission for each change. This is applicable to AI-DSFs that the manufacturer intends to modify over time, including those with automatic (“continuous learning”) or manual modifications.
Details:
If you plan to utilize a PCCP, your marketing submission must include:
- Description of the Modification(s): Clearly define the types of modifications you anticipate making to the AI model or software function based on continued learning or new data. Specify if modifications will be automatic or require human intervention, and if they will be applied globally or locally.
- Modification Protocol: Detail the methods you will use to develop, validate, and implement the proposed modifications. This includes:
- Data management practices: How new data will be managed for retraining, including data provenance, quality checks, and annotation processes.
- Retraining practices: The process for retraining the AI model, including triggers for retraining, model architecture changes, hyperparameter tuning, and version control.
- Performance evaluation protocols: Predefined acceptance criteria and methods for verifying and validating the modifications to ensure continued safety and efficacy. Define metrics, thresholds, and statistical methods.
- Update procedures: How you will update procedures, communicate changes to users, and implement real-world monitoring plans.
- Impact Assessment: Analyze the potential benefits and risks associated with implementing the post-approval modifications and describe how you will mitigate potential risks.
- Scope of Applicability: Understand that the PCCP guidance applies to all AI-DFS, not just machine learning. PCCPs are generally applicable to the device component of combination products.
- PCCP and Submission Pathways: Understand that PCCPs can be used with PMA, traditional 510(k), and De Novo pathways, but not special 510(k) applications. While you can discuss PCCP plans in pre-submissions, the FDA does not formally authorize the PCCP at that stage. The comparison for substantial equivalence in a 510(k) with a PCCP should be to the version of the predicate device before changes made under the PCCP.
The evolving landscape of AI-enabled medical devices demands a thorough understanding of FDA guidance and requirements. Staying compliant while maintaining innovation requires expert navigation of these regulatory pathways.
Need Expert Regulatory Guidance?
Partner with Veranex’s regulatory affairs team to ensure your AI-enabled medical device meets FDA requirements. Our specialists provide comprehensive support throughout the submission process, from early engagement to final approval.
Contact our regulatory experts today to discuss your AI-enabled medical device submission strategy.