As artificial intelligence continues to revolutionize medical device development, the FDA’s evolving regulatory framework presents both opportunities and challenges for manufacturers. The recently released guidance on AI/ML-enabled medical devices introduces crucial requirements that impact development strategies, validation processes, and market entry timelines. At Veranex, our regulatory and AI development teams are prepared to help clients navigate these new requirements while maintaining momentum. This FAQ addresses the most common questions we receive from medical device manufacturers about AI implementation, from Predetermined Change Control Plan (PCCP) requirements to transparency principles that ensure both regulatory compliance and patient safety. Read part one of this series here, and part two here.

FAQ #1: When is a PCCP recommended or required?
Here’s a breakdown of what determines whether a Predetermined Change Control Plan (PCCP) for a Medical Device is relevant and when a manufacturer might choose to include one:
- PCCPs are for Pre-Approving Future Modifications: The primary purpose of a PCCP is to prospectively specify and seek premarket authorization for intended modifications to a device having Artificial Intelligence-Enabled Device Software Functions (AI-DSF). These are modifications that generally would otherwise require a new marketing submission (PMA supplement or new premarket notification).
- Not Required for Initial Authorization: The guidance emphasizes that premarket authorization for an AI-DSF with a PCCP can be established through the standard pathways (PMA, 510(k), or De Novo). The PCCP is reviewed and authorized as part of this initial marketing authorization.
- Manufacturer’s Choice: Including a PCCP is a strategic decision by the manufacturer. They choose to include it if they anticipate making specific types of modifications to their AI-DSF in the future and want to have a pre-approved framework for implementing those changes without needing separate submissions for each one.
- Triggers for Considering a PCCP: A manufacturer would consider including a PCCP if their AI-DSF is designed to:
- Iteratively improve: Where the AI model is expected to be retrained or updated based on new data.
- Adapt to new inputs or environments: Where the device might need to accommodate new data sources or be compatible with different hardware/software.
- Expand its use within defined parameters: For example, extending use to a specific subpopulation.
- What Happens Without a PCCP: If a manufacturer does not include a PCCP in their initial submission, any significant modifications to the AI-DSF that could affect safety or effectiveness would require a new marketing submission at the time the modification is planned.
In summary, a PCCP is not a regulatory requirement for all medical products. It’s a tool available to manufacturers of AI-DSFs that allows them to proactively plan for and obtain pre-authorization for specific future modifications, streamlining the process and potentially reducing the burden of multiple submissions. The decision to include a PCCP is driven by the nature of the AI-DSF and the manufacturer’s strategy for its ongoing development and improvement.
FAQ #2: What are some typical components in the various phases of an AI lifecycle?
Lifecycle Document:
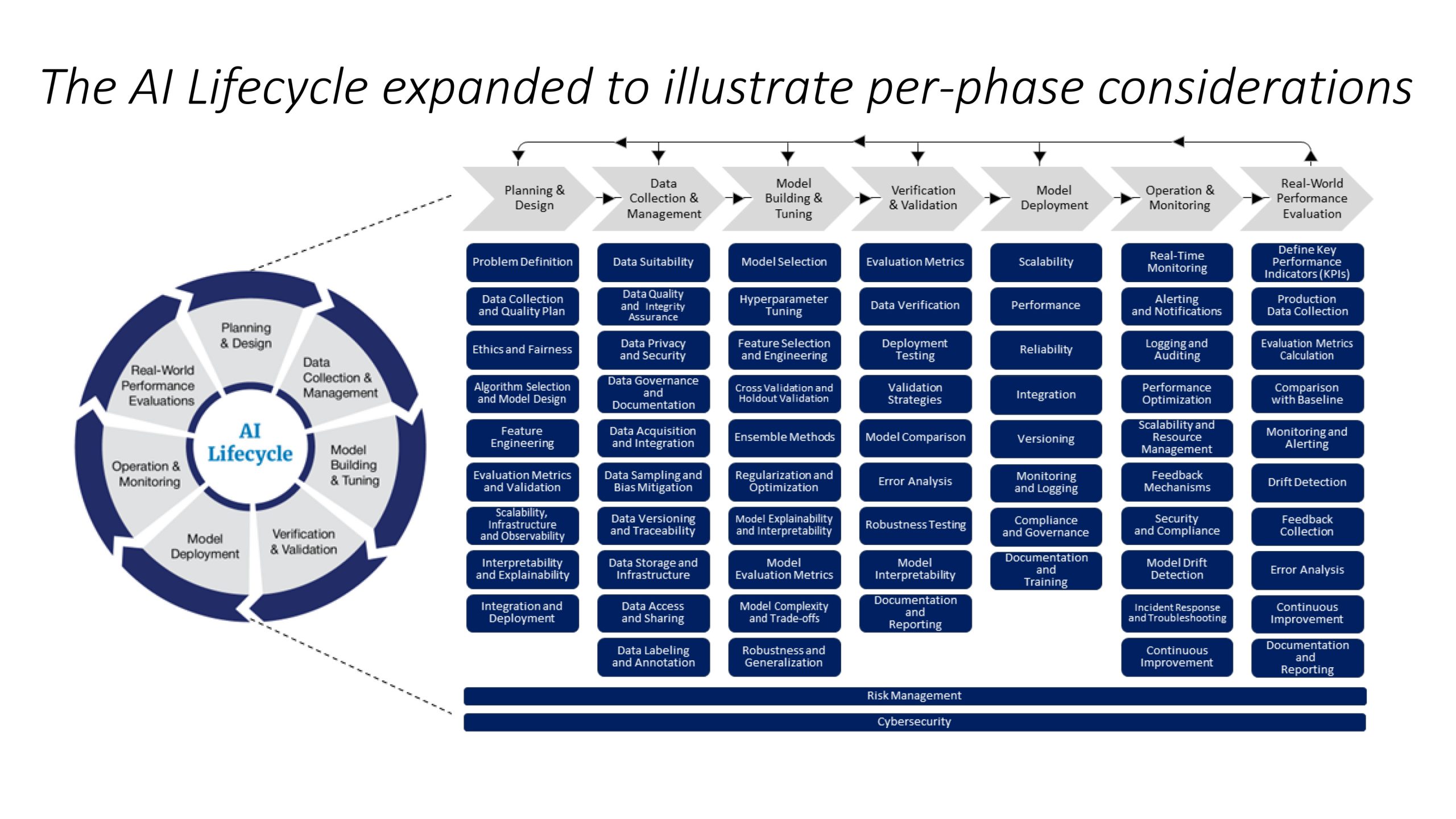
FAQ #3: What are Good Machine Learning Practice (GMLP) Guiding Principles for Medical Devices?
Good Machine Learning Practice (GMLP) Guiding Principles for Medical Devices
These principles are designed to ensure the safety, effectiveness, and high quality of AI/ML-powered medical devices. Keep these in mind throughout the entire product lifecycle:
1. Multi-Disciplinary Expertise:
- Involve experts from various fields (clinical, software, etc.) to understand the clinical workflow, benefits, and risks associated with the device.
2. Good Software Engineering & Security:
- Implement robust software engineering practices, data quality assurance, data management, and cybersecurity measures.
- Use methodical risk management and design processes.
- Ensure data authenticity and integrity.
3. Representative Data:
- Ensure clinical study participants and datasets accurately represent the intended patient population (age, gender, race, etc.).
- Use adequate sample sizes to generalize results.
- Manage bias and assess usability.
4. Independent Datasets:
- Keep training and test datasets completely independent of each other.
- Address all potential sources of dependence (patient, data acquisition, site factors).
5. Best Available Reference Datasets:
- Use accepted, best-available methods for developing reference datasets.
- Understand the limitations of the reference data.
- Use reference datasets that promote model robustness and generalizability.
6. Tailored Model Design:
- Design models that are appropriate for the available data and intended use.
- Actively mitigate risks like overfitting, performance degradation, and security vulnerabilities.
- Ensure the model meets clinically meaningful performance goals.
7. Human-AI Team Performance:
- · When a human is involved, focus on the performance of the entire Human-AI team, not just the model in isolation.
- · Address human factors and the interpretability of model outputs.
8. Clinically Relevant Testing:
- Develop and execute statistically sound test plans using data independent of the training set.
- Test under clinically relevant conditions, considering the intended patient population, subgroups, environment, and potential confounding factors.
9. Clear User Information:
- Provide users (healthcare providers, patients) with clear, contextually relevant information, including:
- Intended use and indications
- Model performance for subgroups
- Data used for training and testing
- Acceptable inputs
- Known limitations
- User interface interpretation
- Clinical workflow integration
- Device modifications and updates
- Means to communicate concerns
10. Performance Monitoring & Retraining:
- Monitor deployed models in real-world use for safety and performance.
- Implement controls to manage risks (overfitting, bias, degradation) when models are retrained after deployment.
Key Takeaways for Developers:
- Focus on the entire lifecycle: From design to deployment and monitoring.
- Data is critical: Ensure data quality, representativeness, and independence.
- Consider the human element: How will the model be used in practice?
- Transparency is key: Provide clear information to users.
- Continuous monitoring: Track performance and manage retraining risks.
This summary should help you quickly grasp the key principles of GMLP for medical devices. Remember to refer to the full document for more detailed information. Credit: https://www.fda.gov/medical-devices/software-medical-device-samd/good-machine-learning-practice-medical-device-development-guiding-principles
FAQ #4: What are the guiding principles related to Transparency for Machine Learning-Enabled Medical Devices?
This is a summary of “Transparency for Machine Learning-Enabled Medical Devices: Guiding Principles“, developed by the FDA, Health Canada, and the United Kingdom’s Medicines and Healthcare products Regulatory Agency (MHRA), that outlines guiding principles for transparency in machine learning-enabled medical devices (MLMDs). It builds upon the previously established Good Machine Learning Practice (GMLP) principles, particularly focusing on the performance of the human-AI team and the provision of clear user information.
The guiding principles are structured around six key questions:
- Who (relevant audiences),
- Why (motivation),
- What (relevant information),
- Where (placement of information),
- When (timing), and
- How (methods to support transparency).
Transparency is crucial for patient-centered care, safe and effective device use, risk management, and promoting health equity. Relevant information includes device characterization, workflow integration, performance, benefits, risks, development, and limitations. Information should be accessible through the user interface, personalized, and delivered at appropriate times.
The following table was extracted from Transparency for Machine Learning-Enabled Medical Devices: Guiding Principles“”, “Table 1: Summary of transparency guiding principles”:
Who: Relevant audiences for transparency |
Transparency is relevant to all parties involved in a patient’s health care, including those intended to:
|
Why: Motivation for transparency |
Transparency supports:
|
What: Relevant information |
Enabling an understanding of the MLMD includes sharing relevant information on:
|
Where: Placement of information |
Maximizing the utility of the software user interface can:
|
When: Timing of communication |
Timely communication can support successful transparency, such as:
|
How: Methods to support transparency |
Human-centred design principles can support transparency. |
Source of above: Transparency for Machine Learning-Enabled Medical Devices: Guiding Principles – June 2024
Regarding Transparency, Appendix B from “Artificial Intelligence-Enabled Device Software Functions: Lifecycle Management and Marketing Submission Recommendations” provides recommendations for designing transparent AI-enabled medical devices, emphasizing a user-centered approach throughout the Total Product Life Cycle (TPLC).
Transparency should be integrated from the initial design phase, rather than as an afterthought, to ensure that relevant performance and design information is clearly communicated to stakeholders in an understandable and actionable way. This involves making information accessible, functionally comprehensible, and connected to the device’s usability.
The design process should begin with a comprehensive understanding of the device’s context of use, including how various factors impact performance. Transparency should be considered across the entire lifecycle, from implementation to decommissioning.
The user interface is a critical area for applying transparency principles, complementing printed labeling with timely and contextually relevant information. This includes alerts, hardware components, and display screens. The design process should identify user tasks, associated risks, and how and when information is needed, integrating risk controls and validating that users can understand the information.
The document emphasizes that transparency is context-dependent, varying based on the device’s risk profile and user needs. It stresses the importance of providing the right information at the right time, focusing on user tasks and the information needed to perform them safely and effectively. This includes considering who needs the information, when they need it, and the context of use.
The user interface should be designed to communicate information effectively through various elements, such as packaging, labeling, training, controls, displays, outputs, alarms, and the logic of operation. Understanding user characteristics, needs, and limitations is crucial, including their functional capabilities, experience, training, and how new information compares to past experiences.
The communication style and format should be clear and appropriate for each user and task, considering factors like reading level, information location, and design elements. Finally, while explainability tools and visualizations can enhance transparency, they must be well-designed and validated to avoid misleading users.
Source: Artificial Intelligence-Enabled Device Software Functions: Lifecycle Management and Marketing Submission Recommendations – Draft Guidance for Industry and Food and Drug Administration Staff (Appendix B)
The Takeaway
Understanding and implementing the FDA’s AI/ML guidance requires a sophisticated blend of regulatory expertise, technical knowledge, and strategic planning. The answers provided here offer a foundation for navigating these complex requirements, but successful implementation demands a partner with deep experience in both AI development and medical device regulations.
Veranex’s integrated approach combines regulatory strategy, AI development expertise, and clinical validation capabilities to help manufacturers navigate these evolving requirements efficiently. Our team’s expertise in implementing Good Machine Learning Practices (GMLP) and managing AI lifecycles ensures that innovative medical devices not only meet regulatory requirements but also deliver meaningful clinical benefits to patients. For more detailed guidance on your specific AI-enabled medical device development journey, contact our team.